안녕하세요 윤도현입니다. 이번 글에서는 Airflow와 IRIS 데이터셋을 이용하여
데이터 수집 → 전처리 → 모델 학습 → 성능 저장까지 자동화하는 방법에 대해 정리하였습니다.
관련글
[2][MLOps] Airflow 2 - 환경세팅(with docker)
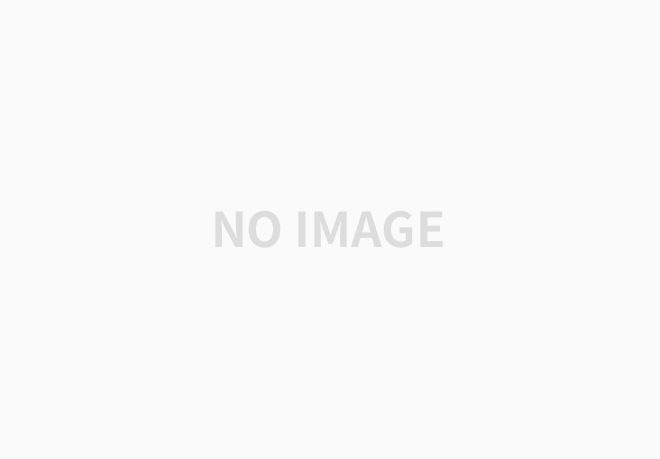
1. 초기 세팅
1.1 Airflow docker-compose.yaml 파일이 있는 디렉토리에 필요한 디렉토리 생성
mkdir -p ./data ./scripts
- data/: 결과 저장용
- scripts/: 전처리/학습 코드 넣는 폴더
1.2 docker-compose.yaml 파일 내 볼륨 마운트 설정
volumes:
- ./dags:/opt/airflow/dags
- ./logs:/opt/airflow/logs
- ./plugins:/opt/airflow/plugins
- ./scripts:/opt/airflow/scripts
- ./data:/opt/airflow/data
# docker compose 재실행
docker compose restart
2. 기본 폴더 구조 설정
airflow-docker/
├── dags/
│ └── ml_pipeline.py ← DAG 파일
├── scripts/
│ ├── preprocess.py
│ └── train.py
3. 스크립트 작성(전처리, 학습, DAG 파일)
3.1 전처리 스크립트(scripts/preprocess.py)
# scripts/preprocess.py
import pandas as pd
from sklearn.datasets import load_iris
def preprocess():
iris = load_iris()
df = pd.DataFrame(iris.data, columns=iris.feature_names)
df["target"] = iris.target
df.to_csv("/opt/airflow/data/iris.csv", index=False)
print("Preprocessing completed.")
3.2 모델 학습 스크립트(scripts/train.py)
# scripts/train.py
import pandas as pd
from sklearn.ensemble import RandomForestClassifier
from sklearn.metrics import accuracy_score
def train():
df = pd.read_csv("/opt/airflow/data/iris.csv")
X = df.drop("target", axis=1)
y = df["target"]
model = RandomForestClassifier()
model.fit(X, y)
acc = accuracy_score(y, model.predict(X))
with open("/opt/airflow/data/result.txt", "w") as f:
f.write(f"Train Accuracy: {acc:.4f}")
print("Training completed.")
3.3 DAG 파일(dags/ml_pipeline.py)
from airflow import DAG
from airflow.operators.python import PythonOperator
from datetime import datetime
import sys
sys.path.append("/opt/airflow/scripts") # 경로 등록
from preprocess import preprocess
from train import train
with DAG(
dag_id="ml_pipeline_dag",
start_date=datetime(2023, 1, 1),
schedule_interval="@daily",
catchup=False,
tags=["ml", "pipeline"]
) as dag:
t1 = PythonOperator(
task_id="preprocess_data",
python_callable=preprocess
)
t2 = PythonOperator(
task_id="train_model",
python_callable=train
)
t1 >> t2 # 전처리 후 학습
4. Dag 실행
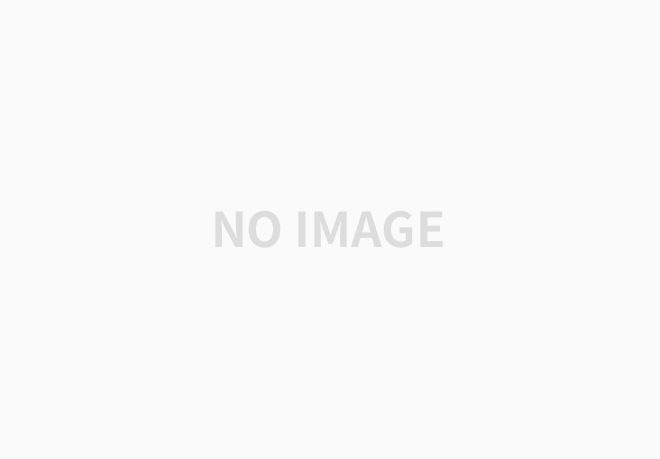
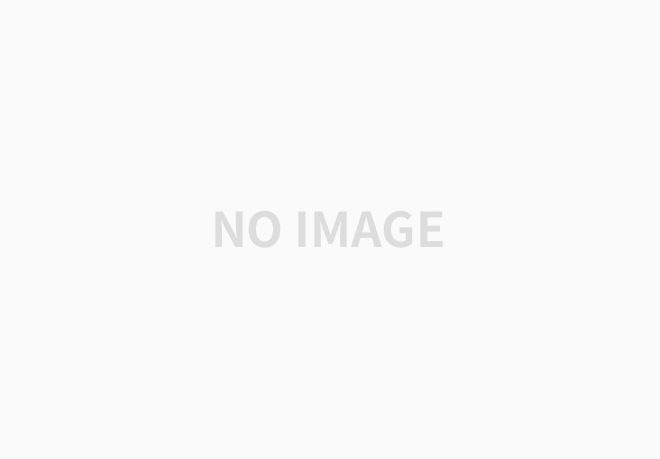
5. 결과 확인(data/result.txt)
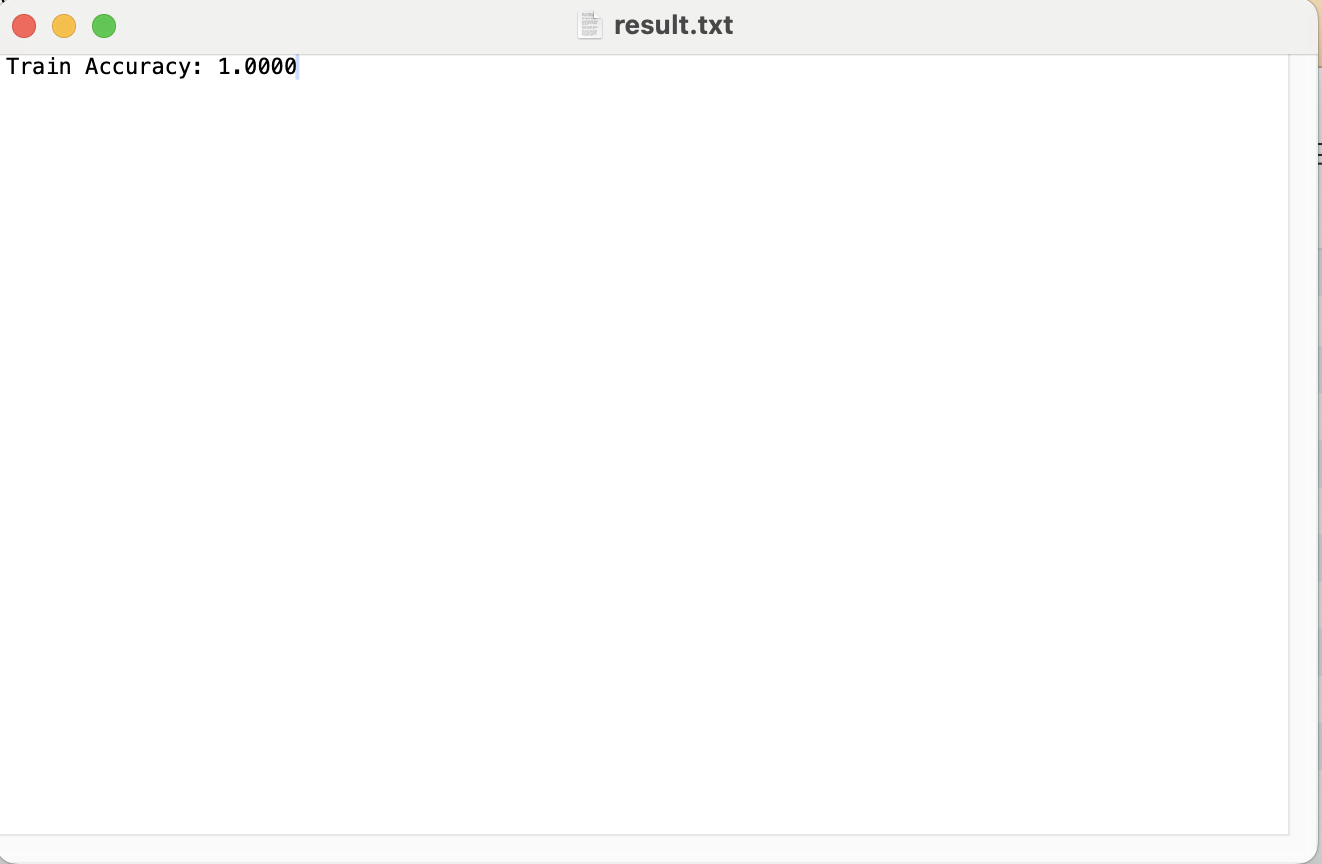
여기까지 Airflow를 이용하여 IRIS 데이터셋을 전처리하고 RandomForest 모델을 학습한 뒤 학습결과를 테스트하는 일련의
워크플로우를 자동화해보았습니다!!!
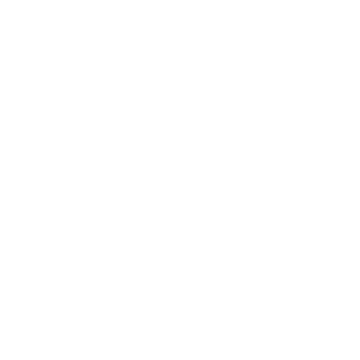
'기본기 > MLOps' 카테고리의 다른 글
[MLOps] Deepstream 3 - 실습(Multi-stream Pose Estimation) (0) | 2025.03.27 |
---|---|
[MLOps] DeepStream 2 - 환경세팅(with docker) (0) | 2025.03.26 |
[MLOps] Deepstream 1 - 기본개념 (0) | 2025.03.26 |
[MLOps] Airflow 2 - 환경세팅(with docker) (0) | 2025.03.25 |
[MLOps] Airflow 1 - 기본개념 (0) | 2025.03.25 |